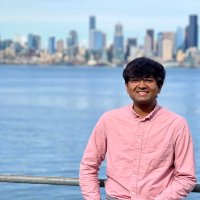
Gantavya Bhatt
@BhattGantavya
Ph.D. Student @UW, MELODI Lab and @uw_wail at @uwcse Formerly @amazonscience, EE undergrad @iitdelhi. An active photographer and Alpinist!
ID:1011498496648548358
https://sites.google.com/view/gbhatt/ 26-06-2018 06:36:49
1,0K Tweets
545 Followers
1,4K Following
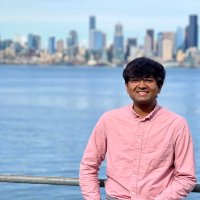
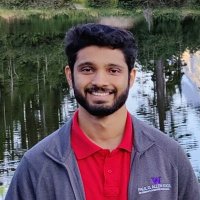
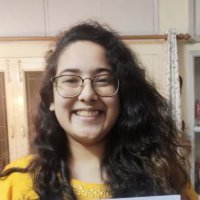
📢 We propose a new benchmark called PhonologyBench for testing how well LLMs perform on three tasks that require sound knowledge : phonemic transcription, counting syllables, and listing possible rhymes.
w/ Harshita Khandelwal, Violet Peng 1/3
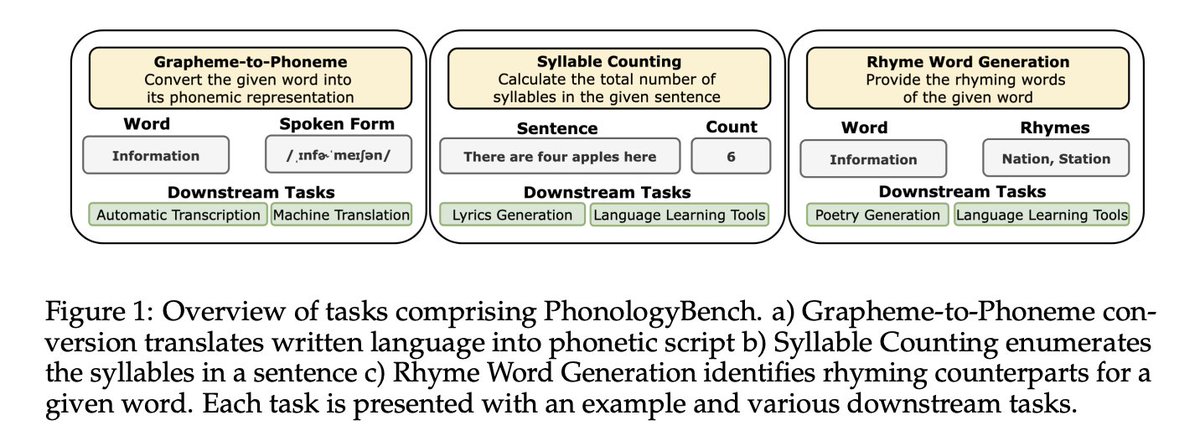
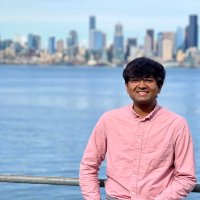
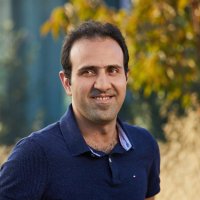
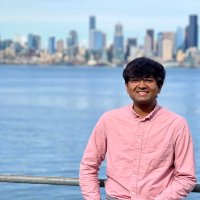
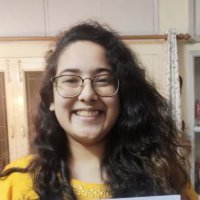
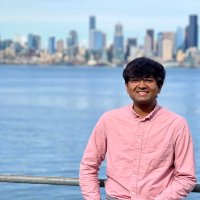







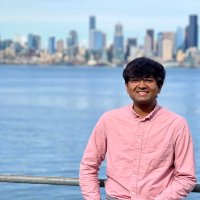
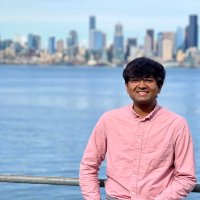
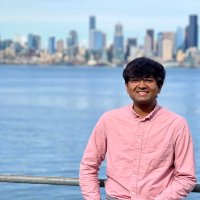
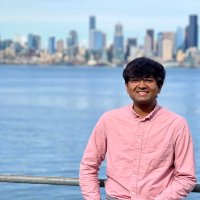
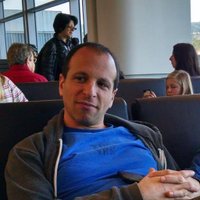