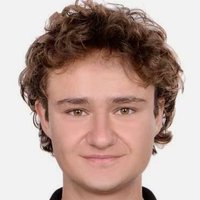
Cem Anil
@cem__anil
Machine learning / AI Safety at @AnthropicAI and University of Toronto / Vector Institute. Prev. student researcher @google (Blueshift Team) and @nvidia.
ID:1062518594356035584
https://www.cs.toronto.edu/~anilcem/ 14-11-2018 01:32:28
450 Tweets
1,5K Followers
1,3K Following
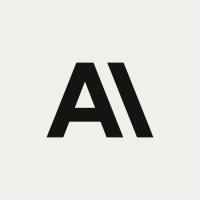

Excited to share Penzai, a JAX research toolkit from Google DeepMind for building, editing, and visualizing neural networks! Penzai makes it easy to see model internals and lets you inject custom logic anywhere.
Check it out on GitHub: github.com/google-deepmin…
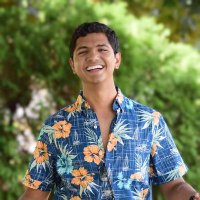
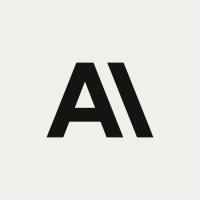
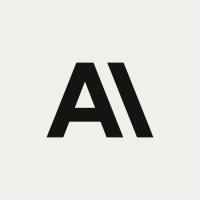
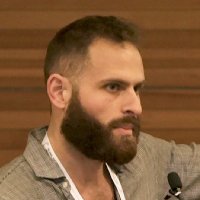
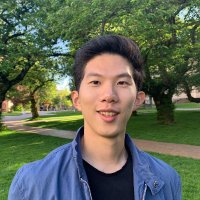
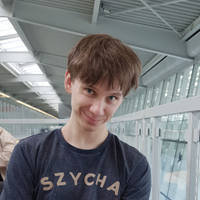
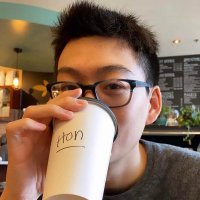
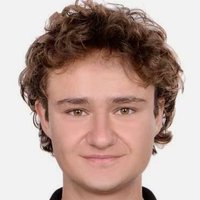
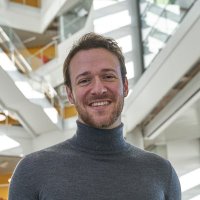

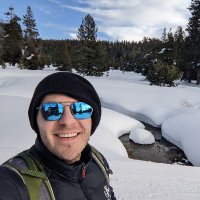
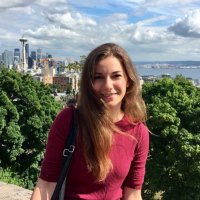
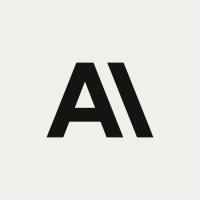

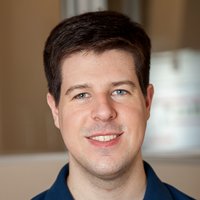
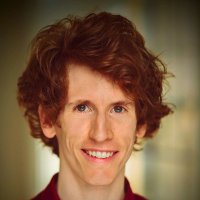